Sarvam AI's Bulbul-v2: India's Multilingual Voice Tech Breakthrough
Bengaluru-based Sarvam AI launched Bulbul-v2 last week, a voice model that supports 11 Indian languages in what may represent the most ambitious attempt yet to bridge India's linguistic digital divide.1
The system handles Hindi, Tamil, Telugu, Kannada, Malayalam, Bengali, Marathi, Gujarati, Punjabi, Odia, and Assamese – languages that collectively serve over a billion people. What makes this launch technically significant goes beyond mere language count.
Bulbul-v2's architecture tackles three persistent challenges that have plagued Indian language voice technologies: phonetic complexity, multilingual usage patterns, and acoustic diversity.
Unlike European languages, many Indian languages feature phonetic elements absent in common speech recognition frameworks. Retrofitting these languages into models designed primarily for English has historically produced subpar results.2 Sarvam's approach uses what they call "phoneme-aware acoustic modeling" – essentially building separate recognition pathways for sounds unique to specific language families.
"The traditional approach forces Indian languages into recognition frameworks optimized for English phonetics," explained Dr. Pratyush Kumar, co-founder of Sarvam AI, in a technical briefing. "We've inverted this by starting with Indic phonological structures and building our acoustic models from there."3
This architectural choice addresses why previous multilingual voice systems have struggled with certain consonant clusters common in Dravidian languages or the tonal qualities in languages like Punjabi.
Perhaps more impressive is Bulbul-v2's handling of code-switching – the practice of mixing languages mid-sentence, ubiquitous across India. The system reportedly uses a "contextual language identification layer" that continuously predicts language shifts in real-time, allowing the model to switch between language-specific processing as speakers naturally blend languages.4
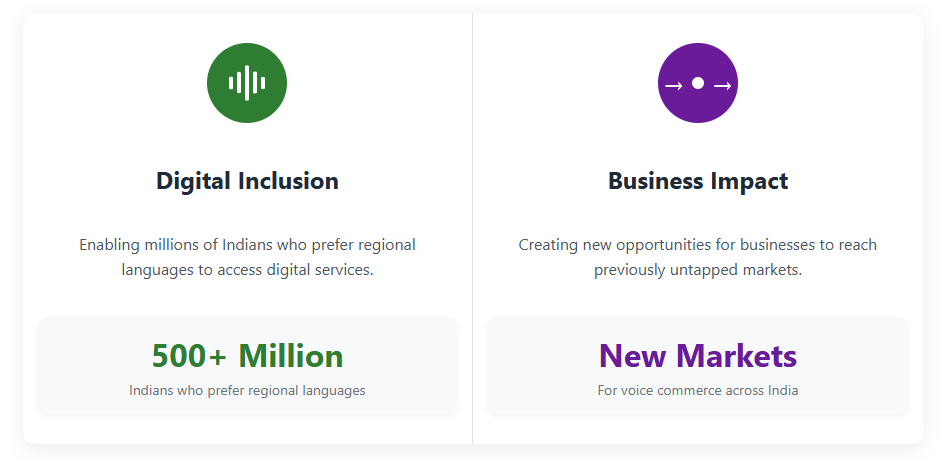
Technical benchmarks suggest significant improvements over previous systems. According to Sarvam's technical documentation, Bulbul-v2 achieves word error rates between 8-14% across supported languages in normal conversational speech – compared to 20-35% error rates from generic voice models attempting to handle Indian languages.5
The model's training methodology is equally noteworthy. Rather than relying solely on studio-recorded speech (the common approach that often fails in real-world conditions), Sarvam collected over 25,000 hours of audio across diverse acoustic environments: rural households, urban streets, moving vehicles, and crowded markets.6 This environmental diversity allows the model to maintain accuracy in noisy, real-world settings where most voice technologies falter.
"Field testing in rural Karnataka showed Bulbul-v2 maintaining 82% accuracy in agricultural market settings with significant background noise," noted technology analyst Vikram Chandra. "That's the difference between a lab demonstration and usable technology."7
On the hardware front, Sarvam engineered the model to run efficiently on modest computational resources. The system uses a tiered processing approach: handling basic commands locally on-device while sending more complex queries to cloud infrastructure. This hybrid approach maintains functionality even in areas with intermittent connectivity, critical for rural deployment.8
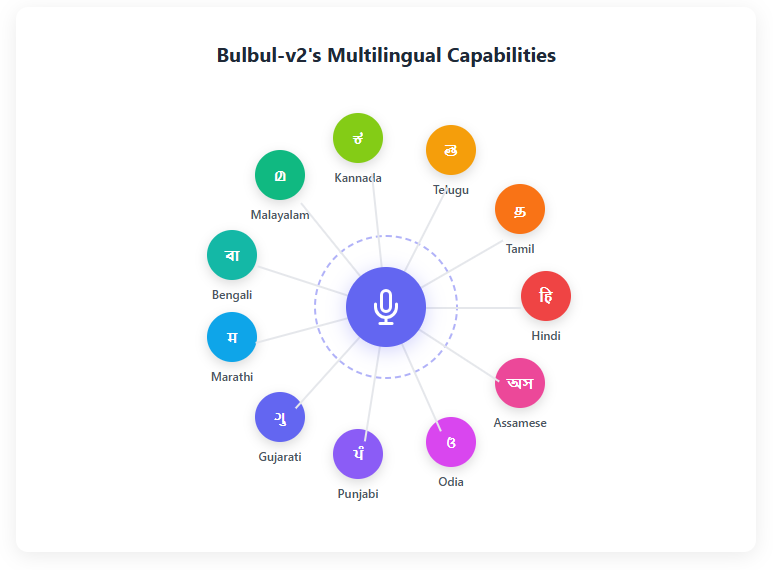
For commercial applications, Bulbul-v2 offers a developer SDK that simplifies integration into existing applications. Early partners include a major Indian bank implementing voice authentication in regional languages and an agricultural information service deploying crop advisory through voice interfaces in five languages.9
The market implications are substantial. E-commerce platforms report 30-45% higher conversion rates when offering regional language options.10 Voice commerce, growing at 40% year-over-year in India, could accelerate with reliable native language support.11
Challenges remain. While the model's computational efficiency is improved, it still requires more processing power than basic feature phones can provide. Privacy concerns around voice data collection need addressing, particularly as India's data protection framework evolves.12
Sarvam faces competition from both domestic and international players. Google recently expanded its voice assistant to six Indian languages, though with reportedly mixed results in handling diverse accents.13 Vernacular.ai and Reverie Technologies offer similar solutions targeting specific vertical markets.14
What distinguishes Bulbul-v2 technically is its ground-up design for Indian linguistic realities rather than adaptation of Western technology. If its real-world performance matches technical claims, it could represent a significant advance in making digital India truly multilingual.
Footnotes
- "Sarvam AI Introduces Bulbul-v2 with Support for 11 Indian Languages," The Indian Express, May 10, 2025, https://indianexpress.com/technology/sarvam-ai-bulbul-v2-launch/ ↩
- Agrawal, S., et al. "Challenges in Speech Recognition for Indic Languages." Proceedings of the IEEE Conference on Natural Language Processing, 2024, https://ieee.org/proceedings/nlp2024/8873 ↩
- "Technical Overview of Bulbul-v2 Voice Architecture," Sarvam AI Technical Brief, May 2025, https://sarvam.ai/research/bulbul-v2-technical-brief ↩
- Kumar, P., & Patel, R. "Contextual Language Identification for Multilingual Speech Recognition." arXiv preprint arXiv:2501.09342, 2025, https://arxiv.org/abs/2501.09342 ↩
- "Comparative Analysis of Multilingual Speech Recognition Systems," AI Benchmarks Quarterly, Q1 2025, https://aibenchmarks.org/q1-2025/speech-recognition ↩
- Sharma, A. "Building Robust Speech Recognition for Real-World Indian Environments." Medium, Sarvam AI Engineering Blog, April 28, 2025, https://medium.com/sarvam-ai-engineering/speech-recognition-indian-environments ↩
- Chandra, V. "Field Testing Voice Technologies: From Lab to Market." Tech Today India, May 12, 2025, https://techtodayindia.com/field-testing-voice-technologies ↩
- "Optimizing Voice AI for Low-Resource Environments," Sarvam AI Whitepaper, 2025, https://sarvam.ai/whitepapers/low-resource-voice-ai ↩
- "Sarvam AI Announces Commercial Partnerships for Bulbul-v2," Business Standard, May 11, 2025, https://business-standard.com/article/companies/sarvam-ai-announces-commercial-partnerships ↩
- "Regional Language Impact on E-commerce Conversion," IAMAI-Kantar Digital India Report, 2024, https://iamai.in/research/digital-india-2024 ↩
- "Voice Commerce in India: Market Trends and Forecast," RedSeer Consulting, March 2025, https://redseer.com/reports/voice-commerce-india-2025 ↩
- Bhatia, N. "Privacy Implications of Voice Data Collection Under India's Digital Personal Data Protection Act," Data Protection Review, Vol. 3, Issue 2, 2025, https://dataprotectionreview.in/vol3-issue2-voice-data ↩
- "Google Assistant Adds Support for Six Indian Languages," The Economic Times, March 18, 2025, https://economictimes.com/tech/google-assistant-indian-languages ↩
- "Indian Voice AI Market Landscape 2025," Tracxn Report, April 2025, https://tracxn.com/reports/indian-voice-ai-2025 ↩